This was meant to be a review of Revolutionary Mathematics by Justin Joque, but it became an essay on one of his points. A friend sent me a great review—so I’m off the hook. Joque’s book examines the radical potential of mathematics to reshape society, critiquing conventional practice and positioning math as a tool for social change. He explores its intersections with culture and activism, urging us to rethink its role beyond traditional frameworks. For me, it sparked deeper questions about thinking itself—how knowledge, data epistemology, and human insight are fundamentally threatened by our growing reliance on the technology of ghostly inference, where intellectual labour is not merely automated but restructured, displacing those who once performed it while subtly embedding the very biases and inequalities it claims to transcend.
Joque’s reference to George Berkeley (March 1685 – January 1753) in his book piqued my curiosity, especially as Berkeley’s critique in The Analyst (1734) challenged the abstract nature of infinitesimals in calculus, an idea that I just re-read in Wittgenstein. These are, essentially, like quarks or clouds—elusive and intangible, but unlike quarks, which we can at least observe through their effects, or clouds that we can still see, the infinitesimals remain purely abstract, with no direct manifestation. Berkeley argued that these unobservable entities lacked connection to the empirical world, undermining their validity. This critique feels remarkably relevant today, especially with the rise of Artificial Intelligence (AI: see note below). As machines increasingly make decisions based on data, the human dimension of intellectual labour risks being diminished to mere computational tasks. Just as Berkeley questioned mathematical abstractions, we must consider the implications of this abstraction on human intelligence in the AI era.
The rise of artificial intelligence (AI) has become one of the defining phenomena of the 21st century, promising to revolutionize intellectual and manual labour across sectors; however, this promise comes with an implicit threat: the displacement of human thought and expertise by computational models, transforming the nature of governance and intellectual work. The increasingly widespread belief in AI as an agent of efficiency and progress echoes earlier philosophical debates about the nature of knowledge, reality, and the human condition. From the critique of metaphysical abstraction in the Enlightenment to contemporary concerns about automation, the tension between human intellect and technological systems is palpable.
Artificial Intelligence in this essay refers to a broad range of technologies, including artificial intelligence (AI), augmented intelligence (AI), large language models (LLMs), and other related computational tools that enhance decision-making, learning, and data processing capabilities. These technologies encompass machine learning, deep learning, and natural language processing systems that assist or augment human intelligence using computer algorithms.
This philosophical concern is rooted in the intersection of metaphysics and epistemology, where Bayesian probability can offer a framework for assessing belief and knowledge. As machines take over decision-making, Bayesian inference could be used to model how human understanding is increasingly reduced to probabilistic reasoning, driven by data rather than lived experience. The concept of “infinitesimals” in Berkeley’s work, too small to observe directly, mirrors AI’s abstraction, with Bayesian probability similarly depending on unseen or abstract factors. Just as Berkeley questioned mathematical abstractions, we must scrutinize the abstraction of human intelligence through AI systems and their probabilistic reasoning.
AI systems, particularly in governance, often prioritize efficiency over nuance, leading to challenges in addressing complex social issues. For example, AI-based predictive policing models aim to reduce crime by analyzing past data to forecast criminal activity. However, these systems can perpetuate biases by over-policing certain communities or misinterpreting patterns. In Canada, this is evident in the overrepresentation of Indigenous communities in crime statistics, where AI-driven policies may misdiagnose the root causes, such as historical trauma or systemic discrimination, instead of addressing the socio-cultural context that fuels these disparities.
The implementation of AI in public service delivery also poses risks of oversimplification, especially when addressing the needs of vulnerable groups. For instance, in Canada, Indigenous communities have historically faced barriers in accessing health care, education, and social services. AI systems may identify general patterns of need based on demographic data, but they often fail to recognize specific local and cultural factors that are critical in understanding these needs. By relying solely on data-driven models, policymakers risk overlooking essential aspects of accessibility, such as language, geography, or traditional knowledge systems, which are integral to Indigenous communities’ well-being. This could lead to recommendations that do not effectively support their unique requirements.
Furthermore, while AI can process vast amounts of data, its inability to understand cultural nuances means that these models often miss the lived realities of marginalized groups. For example, the challenges faced by immigrants and refugees in Canada are deeply rooted in socio-cultural factors that are not always captured in statistical datasets. AI systems designed to assess eligibility for settlement programs or integration services may overlook the role of social capital, support networks, or personal resilience—factors crucial for successful integration into Canadian society. As a result, AI can produce one-size-fits-all solutions that neglect the complexity of individual experiences, further deepening inequality.
These examples underscore the limitations of AI in governance. While AI systems can process vast amounts of data, they lack the cultural sensitivity and emotional intelligence required to address the intricacies of human experience. Human oversight remains crucial to ensure that AI-driven decisions do not ignore the lived realities of marginalized communities, particularly Indigenous peoples and immigrants in Canada. The challenge is not just technical, but ethical—ensuring that AI serves all citizens equitably, taking into account diverse cultural and social contexts. It is essential that AI is integrated thoughtfully into governance, with a focus on inclusivity and the preservation of human agency.
Berkeley argues that these "infinitesimal" quantities, which are too small to be perceived, cannot be validly used in reasoning, as they detach mathematics from tangible reality. For Berkeley, mathematical concepts must be rooted in empirical experience to be meaningful, and infinitesimals fail this test by being incapable of direct observation or sensory experience.
AI has begun to transform the landscape of intellectual labour, particularly in fields that heavily rely on data analysis. Where human analysts once crafted insights from raw data, AI systems now process and distill these findings at unprecedented speeds. However, the value of human expertise lies not only in the speed of calculation but in the depth of context that accompanies interpretation. While AI systems can detect patterns and correlations within data, they struggle to navigate the complexities of the lived experience—factors like historical context, cultural implications, or social nuances that often turn a dataset into meaningful knowledge.
Data analytics, now increasingly dependent on algorithmic models, also underscores this divide. Machine learning can spot trends and produce statistical conclusions, yet these models often fail to question underlying assumptions or identify gaps in the data. For instance, predictive analytics might flag trends in employment patterns, but it is the human analyst who can explore why certain trends occur, questioning what the numbers don’t tell us. AI is exceptional at delivering quick, accurate results, but without the reflective layer of human interpretation, it risks presenting a skewed or incomplete picture—particularly in the realm of social data, where lived experiences are often invisible to the machine.
As AI continues to infiltrate sectors like healthcare, immigration, criminal justice, and labour economics, it is increasingly tasked with decisions that once relied on human intellectual labour. However, these systems, built on historical data, often fail to account for the subtle shifts in context that data analysis demands. Machine learning systems may flag patterns of healthcare access based on prior records, but they might miss changes in societal attitudes, emerging public health challenges, or new patterns of inequality. These are the kinds of factors that require a human touch, bridging the gap between raw data and its true significance in real-world terms.
This shift is also reshaping the role of data analysts themselves. Once, data analysts were the interpreters, the voices that gave meaning to numbers. Today, many of these roles are becoming increasingly automated, leaving the human element more on the periphery. As AI systems dominate the decision-making process, intellectual labour becomes more about overseeing these systems than about active analysis. The danger here is the erasure of critical thinking and judgment, qualities that have historically been central to intellectual work. While AI excels at scaling decision-making processes, it lacks the ability to adapt its reasoning to new, unforeseen situations without human guidance.
As AI continues to evolve, its influence on governance and intellectual work deepens. The history of data-driven decision-making is marked by human interpretation, and any move toward a purely algorithmic approach challenges the very foundation of intellectual labour. The increasing reliance on AI-driven processes not only risks simplifying complex social issues but also leads to the marginalization of the nuanced understanding that human intellectual labour brings. This tension between machine efficiency and human insight is not merely a technological concern but a philosophical one—a challenge to the nature of work itself and the role of the intellectual in an age of automation.
This shift invites a reconsideration of the historical context in which intellectual labour has developed, a theme that is crucial in understanding the full implications of AI’s rise. The historical evolution of data analysis, governance, and intellectual work has always involved a negotiation between human cognition and technological advancement. As we look toward the future, we must ask: in an age increasingly dominated by machines, how will we ensure that human experience and judgment remain central in shaping the decisions that affect our societies? This question points toward an urgent need to ground AI in a historical context that recognizes its limitations while acknowledging its potential.
As AI becomes more central in shaping political and social policies, particularly regarding immigration, there are concerns about its ability to reflect the complex realities of diverse communities. The reliance on AI can lead to oversimplified assumptions about the needs and circumstances of immigrants, especially when addressing their integration into Canadian society. AI systems that analyze immigration data could misinterpret or fail to account for factors such as socio-economic status, cultural differences, or regional disparities, all of which are critical to creating inclusive policies.
This evolving landscape signals a deeper erosion of the social contract between Canadians and their governments. In immigration, for example, particularly in light of the 2023–2026 Data Strategy and the findings of CIMM – Responses to the OAG’s Report on Permanent Residents, ensuring human oversight becomes increasingly crucial. Without it, there is a risk of diminishing the personal, human elements that have historically been central to governance. The shift towards automated decision-making could alienate citizens and weaken trust in political institutions, as it overlooks the nuanced needs of individuals who are part of the democratic fabric.
AI’s increasing role in governance marks a shift toward the disembodiment of knowledge, where decisions are made by abstract systems detached from the lived experiences of citizens. As AI systems analyze vast amounts of data, they reduce complex human situations to numerical patterns or algorithmic outputs, effectively stripping away the context and nuance that are crucial for understanding individual and societal needs. In this framework, governance becomes a process of automating decisions based on predictive models, losing the human touch that has historically provided moral, ethical, and social considerations in policy formulation.
The consequences of this abstraction in governance are far-reaching. AI systems prioritize efficiency and scalability over qualitative, often subjective, factors that are integral to human decision-making. For example, immigration decisions influenced by AI tools may overlook the socio-political dynamics or personal histories that shape individuals’ lives. When policy decisions become driven by data points alone, the systems designed to serve citizens may end up alienating them, as the systems lack the empathy and contextual understanding needed to address the full complexity of human existence. This hollowing out of governance shifts power away from human oversight, eroding the ability of democratic institutions to remain responsive and accountable to the people they serve.
The COVID-19 pandemic served as a catalyst for the rapid integration of AI in governance and society. As governments and businesses shifted to remote work models, AI tools were leveraged to maintain productivity and ensure public health safety. Technologies like contact tracing, automated customer service bots, and AI-driven health analytics became critical in managing the crisis. This acceleration not only enhanced the role of AI in public sector decision-making but also pushed the boundaries of its application, embedding it deeper into the governance framework.
The pandemic also saw the domestication of AI through consumer devices, which became central to everyday life. With lockdowns and social distancing measures in place, reliance on digital tools grew, and AI-powered applications—like virtual assistants, fitness trackers, and personalized recommendation systems—found a more prominent place in households. These devices, which had once been seen as niche, became essential tools for managing work, health, and social connections. The widespread use of AI in homes highlighted the shift in governance, where decision-making and the management of societal norms increasingly came under the control of automated systems, marking a techno-political shift in how people interact with technology.In revisiting Berkeley’s critique of infinitesimals, we find philosophical parallels with the rise of AI. Berkeley questioned the very foundation of knowledge, suggesting that our perceptions of the material world were based on subjective experience, not objective truths. Similarly, AI operates in a realm where data is processed and interpreted through systems that may lack subjective human experience. AI doesn’t “understand” the data in the same way humans do, yet it shapes decision-making processes that affect real-world outcomes, creating an abstraction that can be detached from human experience.
This disconnection between machine and human experience leads to the dehumanization of knowledge. AI systems operate on algorithms that prioritize efficiency and optimization, but in doing so, they strip away the nuanced, context-driven understanding that humans bring to complex issues. Knowledge, in this sense, becomes something disembodied, divorced from the lived experiences and emotions that give it meaning. As AI continues to play a central role in governance, the process of knowledge becomes more mechanized and impersonal, further eroding the human dimension of understanding and ethical decision-making. The philosophical concerns raised by Berkeley are mirrored in the ways AI reshapes how we conceptualize and act on knowledge in a tech-driven world.
The rapid integration of AI into intellectual labour and governance presents a profound shift in how decisions are made and knowledge is structured. While AI offers the promise of efficiency and precision, its growing role raises critical concerns about the erosion of human agency and the humanistic dimensions of governance. As AI systems replace human judgment with algorithmic processes, the risk arises that complex social, political, and ethical issues may be oversimplified or misunderstood. The hollowing out of governance, where decision-making is increasingly abstracted from lived experiences, mirrors the philosophical critiques of abstraction seen in Berkeley’s work. The human element, rooted in experience, judgment, and empathy, remains crucial in the application of knowledge. Without mindful oversight, the adoption of AI in governance could result in a future where technology governs us, rather than serving us. To navigate these challenges, preserving human agency and ensuring that AI tools are used as aids rather than replacements is essential to maintaining a just and ethical society.
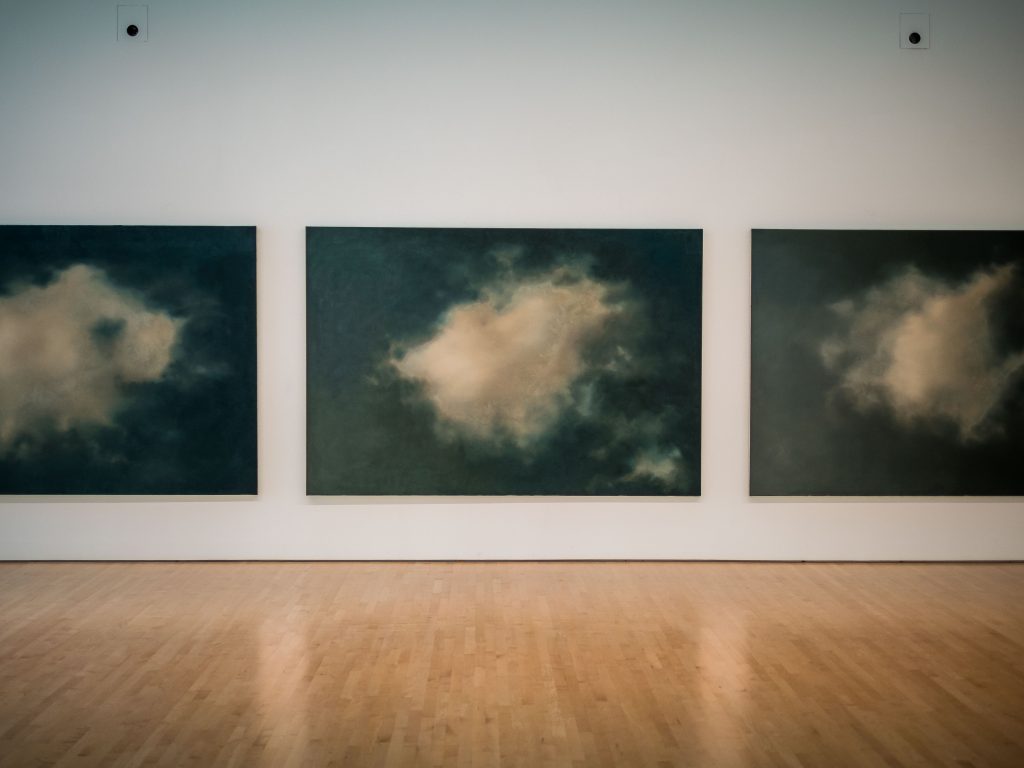